Applied AI Consulting
Improve your products and streamline your workflows with AI and ML to achieve higher quality results efficiently and cost-effectively.
With over 20 years of experience in AI, ML, and Natural Language Processing (NLP), I bring an analytic, process-oriented mindset to automating data-driven marketing and business processes.
Whether you aim to enhance customer experience, boost employee efficiency, streamline company information access, or automate processes, our collaboration ensures you avoid issues by having a clear vision of what to build and how to build it
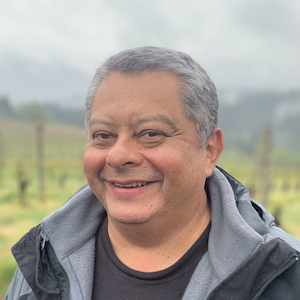
How I can help:
- High Level Strategic Planning - I'll help you cut through the confusing landscape of implementing an AI enabled project to ensure your plan will meet your goals and metrics.
- Comprehensive Guidance. - I'll meet reguarly with your CTO, architect and team lead to review the plans, address challenges that come up and provide advice, insights and training as appropriate.
- Proof of Concept (POC) - After reviewing a project goal and exploring options that may meet the desired outcomes, I'll implement a POC to get more information on how well we can expect a system to perform in production.
No matter your needs, you'll receive personalized attention and solutions tailored to your business goals. Whether you’re a small or medium-sized business owner, I can help you implement AI and ML solutions to elevate your business to the next level.
Julio's extensive and practical knowledge of applied AI is invaluable, especially in the early stages of architecting GenAI/AI solutions.
Julio listens carefully to your vision and takes the time to deeply understand all of the moving parts of your project so he can make targeted recommendations about how to use AI tools to bring it to fruition. ... [He] is the first step to understanding the landscape of possible AI solutions." -
Julio is a developer who fully understands uses of language models, construction of language models and implementation. Now that OpenAI is mainstream he’s a go-to guy for showing a business how they can leverage AI. ... his deep knowledge about the subject matter, his ability to demonstrate and his organization.
Want to find me on the internet? Get in touch at LinkedIn and Twitter